Machine learning is, at its core, all about using data in order to remain ahead of your customers'
furniture buying behaviors.
Machine learning is poised to be the Next Big Thing for retail furniture stores. It promises to revolutionize our business. Whether this is an overblown promise or one that will be fulfilled, one thing is for sure – it’s worth taking a look at. After all, machine learning is, at its core, all about using data in order to remain ahead of trends in customer behavior. Remaining a step ahead of one’s customers is crucial for retailers who strive to always give them the products that customers want. Keeping this in mind, let’s have a detailed look at how machine learning could impact your retail operations.
What is Machine Learning?
In a nutshell, machine learning is a technology that makes it easier to take advantage of big data. Big data refers to enormous and complex data sets that cannot be dealt with using traditional data processing applications. In the past, only the largest most tech savvy companies could sort through and analyze big data in order to glean insights about trends.
However, the emergence of artificial intelligence (AI) and machine learning changed everything. AI is basically the ability of a computer to imitate human logic in order to make decisions. Machine learning refers to the way a computer can learn rules of human logic without being specifically programmed to carry out actions in a certain way. Through machine learning, a computer can constantly improve its understanding of those rules.
All of this may sound like information that is only relevant in a tech context, but there is so much more to it. With the internet creating more data than ever before, the implications of machine learning in retail has become significant, as we will explore below.
Machine Learning and Retail
If there’s one thing that remains a constant in retail, it’s the struggle to make predictions that help increase returns and curtail spending. Traditionally, these predictions were made using methods like linear regressions that would plot sales volume against variables. However, since the human brain is obviously limited in its ability to select and analyze data, this approach can only work for a data set that is appropriately sized.
But once we add machine learning to the mix, everything changes. Machine learning is immeasurably more powerful than historical approaches. That's because machines are able to analyze much larger sets of variables in order to determine the most significant factors, and to make predictions.
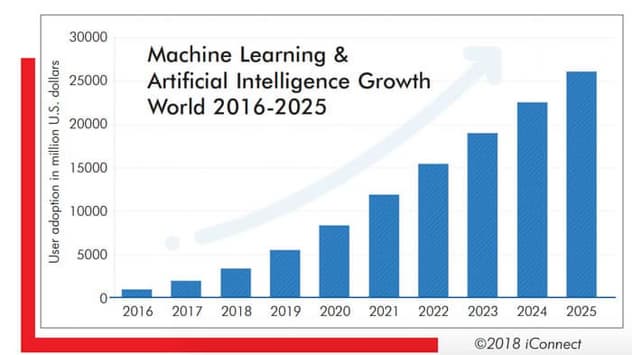
Not only can big data be analyzed using machine learning, but the accuracy of predictions improves. This is because customized models can be adapted for any specific category of business. Today, customized models made possible by machine learning, produce granular, useful information. Specifically, information that can be used to understand and predict the behaviors of target retail buyers. It isn’t enough to simply rely on their past behavior, because customer behavior changes over time.
What machine learning does is discover underlying patterns behind the data. It combines market trends with customer data, providing retailers with action plans that are holistic enough to improve targeting. In doing so, they also make the data actionable by incorporating predictive analytics. Instead of just looking at customers’ past purchases, machine learning enables retailers to plan offerings in a way that provides customers with what they want before they even realize they want it. The ultimate goal of machine learning for retailers is to drive revenue growth. It makes hyper-personalization and better business decision-making possible.
Seven Ways Machine Learning Improves Retail
1. Recommends additional customer buys: When Amazon users buy one item, they are automatically shown additional products other customers tend to purchase at the same time. When it comes to machine learning at retail, Amazon is a pioneer.
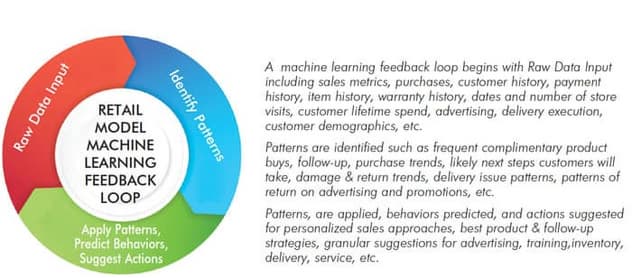
Through the application of data correlation techniques on its enormous customer database, Amazon gains insights into customer preferences, reviews, product popularity and past purchases. It then uses those insights to make personalized and relevant recommendations to match users' preferences and buying history. Amazon analyzes identifying patterns and purchase behaviors on every single product page in order to identify which products tend to be bought together. This allows them to display complementary products at the right time.
2. Recognizes customers: Machine Learning algorithms use Artificial Intelligence to recognize faces from a large set of face images recorded in a store; the technology is able to make decisions based on facial recognition. It can identify sales people, owners, managers and even mail carriers, distinguishing them from buyers. The technology allows furniture retailers to identify buyers by name and track them across their complete purchase life-cycle.
3. Boosts retailer customer retention: Most retailers invest time and effort to attract repeat customers since it’s cheaper to retain customers than acquire new ones.
Advances in the technology allow retailers to identify customers who seem to be about to defect from the brand, and engage with them.
A recent retail case study found that Machine Learning technology effectively used the following variables to bring repeat customers back into a retail store:
- Dates of first and last purchase over the last 5-8 years.
- Number of days between the first and last purchase.
- Total number of sales orders.
- Total number of sales orders for each customer.
- Geography of purchase, by zip when possible.
- Number of items purchased.
- Type of payment or financing.
- Number of visits to the store.
After a few iterations to get the algorithm to predict correctly, the retailer found that 42 percent of its customers were more than likely to fall off the grid, and that sending periodic, highly personalized offers by email from their CRM system was effective in bringing 50 percent of them back into the store once more for a productive 2017 winter. This is just one example of how predictive analysis can increase the probability of a conversion.
4. Increases the average customer spend: Machine learning allows even small retailers to do what once only a behemoth like Amazon could: gain insight about product purchase patterns among their own products. Basically, this means that machine learning allows them to predict a customer’s next most likely move by recognizing products that tend to be purchased together or sequentially, and trigger a follow up purchase in the short term.
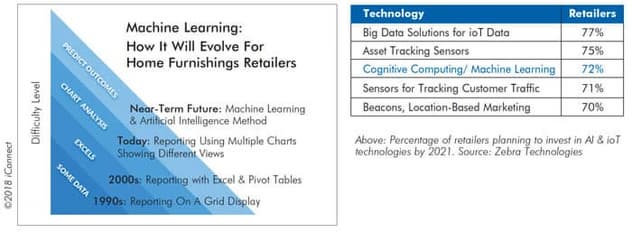
Through continuous analysis of customer demographic data, machine learning understands the buying trends rampant in a particular subset. Not only are similar products tracked, but retailers can generate lists of customers who have the potential to buy locked up inside of them. They can then offer incredibly personalized product recommendations, better target their advertising, promotions, and even personalize individual online experiences based on purchasing history. In addition to boosting revenue, this provides competitive advantage by enhancing the overall customer experience.
The same retailer in the case study described above, adopted Machine Learning to provide sales people, with suggested real-time offers and product suggestions based on a customer's personalized data and what similar customers previously purchased.
5. Enhances cross-selling and up-selling: Through machine learning, retailers can offer the best possible prices to customers based on past purchases. It can even allow retailers to recognize when a customer might need to purchase a product again (for example a mattress or sofa) and send targeted promotions or information based on a customer's age or lifestyle. Today's buyers have high expectations for personalization from retailers. Through machine learning, it is possible to fulfill these expectations and help customers remain loyal.
6. Cuts back on waste: Machine learning algorithms allow retailers to predict the most relevant time to offer products, instead of wasting marketing dollars when the likelihood of purchase is low. In high-volume stores, it can also help retailers decide just how much inventory to stock at a particular time when compared to the rest of the year. By reducing spoiled inventory and unnecessary marketing campaigns, machine learning allows waste to be reduced.
Conclusion
Machine learning helps retailers to understand customer behaviors, which allows them to make
decisions that can grow revenue. By understanding why customers act the way they do, behavior can be influenced. It also allows for operational efficiency that directly affects the company’s bottom line.
Ultimately, machine learning at retail is meant to drive revenue growth efficiently. Using big data based on demographics, individual customers can be given more personalized care. Analyzing large data sets more accurately to inform business decisions helps retailers to constantly update their revenue potential and increase their share-of-wallet.
Amitesh Sinha is a technology consultant based in North America. With over 20 years of experience developing and deploying solutions for retail, Sinha has gained a reputation for home furnishing software solutions, furniture software, POS furniture software, and re-engineering of software with extended features. His company, iConnect offers business technology solutions that integrate with most P.O.S. systems to make them more efficient and user-friendly. For more information about this article or any retail technology question contact Amitesh at 703-471-3964, amitesh@iconnectgroup.com or www.iconnectgroup.com.