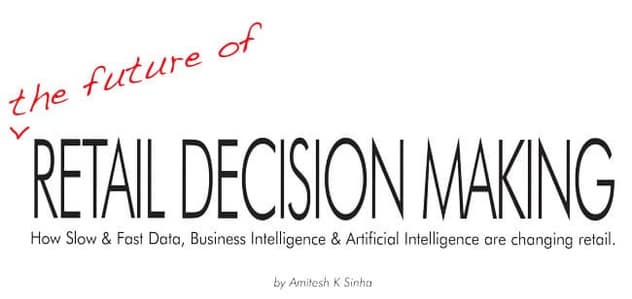
Most home furnishings retailers desperately need business intelligence, their path to future success.
Business acumen has always ruled the market, but where it was a question of shrewdness and intuition before, it is now a scientific field called Business Intelligence that is using innovative technology to replace guesses in predicting and leveraging customer behavior.
Here is a look at the emerging tools and trends of Business Intelligence, and how they may serve the furnishing industry. This may sound intimidating, but it isn’t, especially if you have a person or partner dedicated to creating, and analyzing outputs that can help you be just as smart as the larger retailers, or even smarter than your competition.
Fast & Big Data
In this technological age, we’re used to thinking about data as being fast and big. But there are actually two different approaches to utilizing data.
First, let’s look at the Fast Data approach, with its emphasis on handling data in motion: data that is constantly flowing in from millions of endpoints such as online statistics (Google analytics, etc.), stock management software, in-store customer flow analysis (Heat maps that show where consumers flow and congregate in your store), point of sale software, and much more. The input is then analyzed and acted upon, often within milliseconds.
The Big Data approach relies on the deep analysis of huge amounts of data, mining it for connections, repetitions, and trends, and helping to understand and even predict client behavior.
Fast and Big Data work hand in hand to optimize sales, merchandising and marketing. Fast Data’s split-second decisions are based on the reasoning and trends found via Big Data analysis. And once it’s processed and acted upon at the front of business, Fast Data can then be exported into longer-term storage systems and processed as Big Data.
The pursuit of Big Data in itself is no longer sufficient, because without the acquisition and leveraging of Fast Data, the patterns that emerge out of deep learning algorithms become outdated before they can be applied to the field. On the other hand, Fast Data in itself lacks the deeper insight accrued via Big Data; without Big Data to support it, Fast Data might become direction-less and as irregular as human intuition.
The loop is one of infinite feedback and evolution: scan, act, evaluate, improve the action, scan, act, evaluate, and so on.
Business Intelligence at the End-User Level
While BI systems are written by Information technology (IT) people, their end users are in fact people just like you: executive members of the retail world, Owners, Managers, Marketers & Salespeople. This gap between creators and users is one of the top challenges of efficient, cost-effective BI systems.
For example, IT people are enamored with the ability to drill down to any possible level of resolution. However, for a home furnishing manager, the ability to see if a specific sales team is meeting its daily objectives can be distractive and complicating rather than helpful.
The challenge, then, is for the IT team to fully understand the specifics of every level of management, and the precise questions each manager must answer in order to perform his work. The more senior the manager, the more global and advanced should be the view he receives from the system, without delving into the finer details. Other members of the management team may want more finite information that relates to individual sales performance, merchandising and more. The goal of a good BI application is not to reflect data. It is to lay out this data in insightful ways that will help managers solve problems and advance strategic causes. To that end, BI applications must be as friendly and intuitive as possible. Otherwise, they will raise the resistance of their end users, which might lead to ineffectual use of the system or flat out rejection of it.
Consolidation from Multiple Data Sources
BI-savvy retailers may rely not on one, but on several BI products and applications. Consolidating various input flows into a single, insightful look of the company is a real challenge, but also key in providing higher value.
There are several factors to consider when consolidating BI data:
- Choosing a consolidation solution that will cause minimal loss of data, while ensuring that the aggregated data is searchable, readable, actionable, and capable of providing insight.
- Creating a single unified, precise terminology across various systems, so that each term has a single definition and data collected under each term is in fact compatible.
- Curating data and quality-assurance of the data before consolidating. Redundant or mis-configured data streams might only complicate the system, slow it down, and even cause false-positive recognition of patterns that are not truly there.
- Database hierarchy and data flow direction. It’s impossible to use all BI products as the aggregating entities; instead, there must be a single aggregate solution at the top being fed by the lower databases.
- Data formats must mesh seamlessly, otherwise data might be truncated or corrupted in transfer.
- Security of data transfer between the BI products.
- Automation of the consolidation process for ongoing regular workflow.
- Error handling at the aggregate level and trickling down to the other BI solutions.
Consolidating data, when done correctly, will allow you to compare the three chairs in one system with the two tables in another, and come out with a dining room instead of a logic error. The key to a successful BI platform must revolve around data being accumulated instantly and formatted in an easy to read dashboard that you can access hourly, daily, weekly, etc.
Quality over Quantity
The number of factors that can be scanned, tracked, processed, and stored in BI systems is near infinite. In order to mine reason and insight out of data, that data must be of high quality: clear input correctly measured across various posts and without internal conflict.
If the data you are receiving is of lower quality, its effects on the analysis process can be destructive. Otherwise, the noise that is created and introduced into the system might interrupt the recognition of patterns or even cause false patterns to emerge where they are not in fact there.
Artificial Intelligence (AI) vs. Business Intelligence (BI)
AI attempts to automate the decision making processes that BI people usually do. Are the two disciplines friends or foes, allies or competitors?
AI in basic forms has already taken root in the realm of operational BI and decision management. Quick-learning algorithms are being routinely used to optimize everything from stock management to staff positioning and workload. As AI grows cleverer, the types of problems it can solve grows more complex and rich. Slowly it is crawling up the command chain toward top-level BI decisions.
Consolidation BI
Once you have segregated expense data, purchasing data, customer service data, financial data or inventory data, this information can provide valuable insights into different “singular” areas of your business – but they are not useful in isolation. They will provide you specific knowledge areas of your business, but not in totality because they don’t provide complete insight about every aspect of your business. These elements in your business are all interconnected. Therefore, to help your decision-making skills, you need to consolidate business intelligence from multiple sources and present it in a coherent way, preferably a single dashboard where you can quickly visualize critical elements you need to address. Then you can drill down further for more meaningful analysis and insights.
An Example
You know the furniture item that sells most at your store... Thumbs up!
You know the furniture item that is the least popular among your customers... Thumbs up again!
Analysis in isolation will lead you to decide to continue promoting furniture items that generates the most revenue while moving the other to the warehouse or clearance – Thumbs down!
A consolidated analysis, however might help you make the more profitable decision to create a promotion in which you bundle your most popular product with your least popular furniture item.
Hidden Costs & Opportunities
Let’s look at another example.
You have a furniture retailing chain and you have a supreme belief on your suppliers who provide you furniture at the best possible price. After all what else does a furniture retailer want? A perfect example of, buying low and selling high.
That is until you decide to have a closer analysis, and you come across hidden costs like:
- Excessive labor costs of removing the packaging.
- High cost incurred in moving the item between different distribution centers that in turn invite the risk of damages.
- Problems with prepping and Loading the wrong pieces of furniture a day before delivery.
- Routing the wrong furniture and then have more hands on the merchandise to move it from the delivery bay to layaways or back to the racks.
The proper use of data makes you smart and more profitable, ignoring its uses and the assets you can derive from it, makes you vulnerable and less profitable.
Amitesh Sinha is a technology consultant based in North America. With over 20 years of experience developing and deploying solutions for retail, Sinha has gained a reputation for home furnishing software solutions, furniture software, POS furniture software, and re-engineering of software with extended features. His company, iConnect offers business technology solutions that integrate with most P.O.S. systems to make them more efficient and user-friendly. For more information about this article or any retail technology question contact Amitesh at 703-471-3964, amitesh@iconnectgroup.com or www.iconnectgroup.com.